Prof. Gabriele Pannocchia, UNIPI
March 22, 2024
General overview
One of the core activities of the project is to promote excellence in research through scientific publications regarding automatic control methods and applications oriented to smart industries. In compliance with the EU open-access/open-research policies, all publications arising from the project are available on the Zenodo repository (https://zenodo.org/communities/frontseat/).
During the first half of the project, 36 publications (9 journal papers and 27 conference papers), and 7 posters arose. Publication venues include a mixture of theory- and application-oriented top-quality journals, and the most important conferences in the field of automatic control, such as the IFAC World Congress 2023 held in Yokohama, Japan. Several other conference papers have been accepted for the upcoming conferences this year, such as ADCHEM2024 and NMPC2024.
Major topics covered in these publications are:
- Real-time optimization): theory and applications
- Performance monitoring of industrial control systems
- Model predictive control (MPC): theory and efficient/embedded implementation
- Research- and education-oriented hardware and software for control engineering
- Machine learning methods for optimization-based control system design and monitoring
- Data-driven modeling and control systems design
- Experimental validation of control systems
- Inferential/Soft-sensors and their industrial application
- Cyber-secure process control systems
Highlights
Six publications are here selected and briefly reviewed to showcase the importance of the investigated topics and testify to the fruitful cooperation among the various FrontSeat partners.
1. Iterative real-time optimization of a membrane pilot plant
(https://zenodo.org/records/10826082)
This paper, published in Control Engineering Practice (Elsevier), details the practical application and validation through experimentation of iterative real-time optimization (RTO) within a membrane separation pilot plant operating continuously. The primary aim is to attain economically optimized operations by adjusting the transmembrane pressure and feed flow rate, through setpoints for the lower-level plant controllers. Utilizing a simplified model and modifier-adaptation techniques, the experimental findings demonstrate that despite significant disparities between the actual plant and the model, the introduced RTO approach effectively enhances process economics.
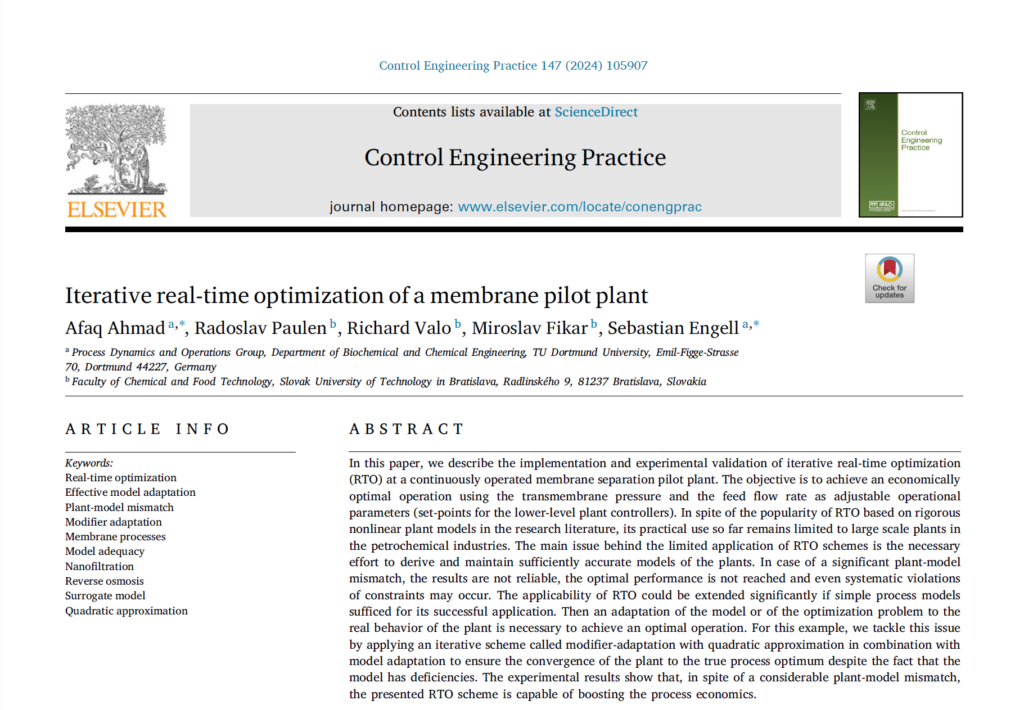
.
2. Data-Based Design of Multi-Model Inferential Sensors
(https://zenodo.org/records/8217445)
The focus of this paper, published in Computers and Chemical Engineering (Elsevier), revolves around the challenge of designing inferential (soft) sensors. The objective is to enhance the predictive capabilities of these sensors while retaining their linear structure. Introducing a direct solution, the paper proposes two innovative methods for constructing multi-model inferential sensors. To illustrate the efficacy of these techniques, inferential sensors are specifically designed for an industrial Vacuum Gasoil Hydrogenation unit. Comparative analysis against several single-model inferential sensors and the existing refinery inferential sensor demonstrates significant advantages of multi-model techniques over current state-of-the-art design methodologies.
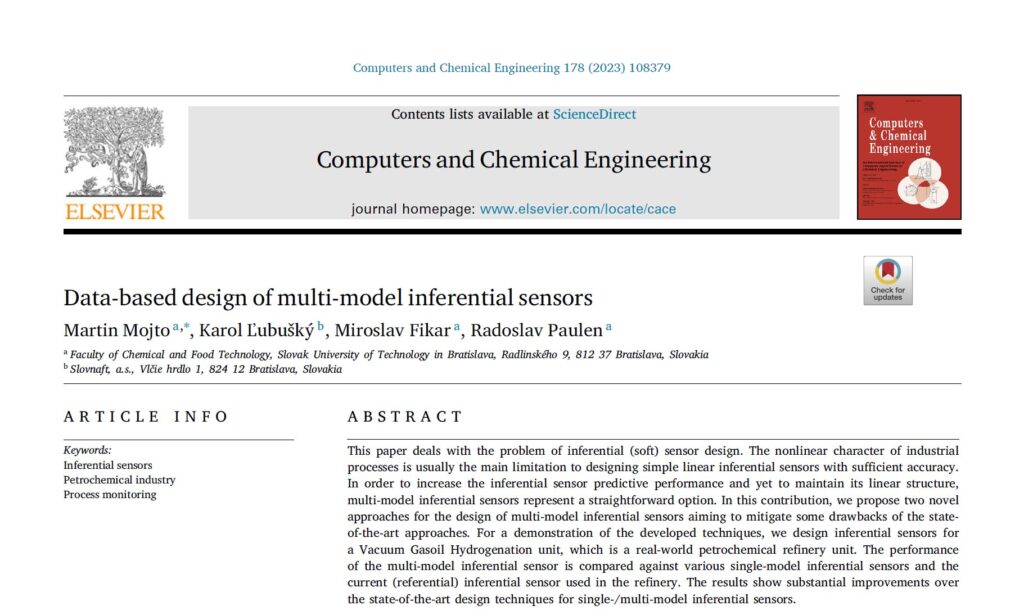
.
3. Exploiting symmetries in active set enumeration for constrained linear–quadratic optimal control
(https://zenodo.org/records/7857174)
Published in Automatica (Elsevier), this research delves into symmetric constrained linear–quadratic optimal control problems and their parametric solutions. Such problems entail finding piecewise affine feedback laws, expressed equivalently as sets of active sets. The study demonstrates that the symmetries inherent in the optimal control problem also manifest in the active sets, facilitating a substantial simplification in identifying these sets. Notably, this paper enhances a recently introduced method for dynamic programming-based enumeration of all active sets, showcasing a significant reduction in computational effort through an illustrative example borrowed from existing literature.
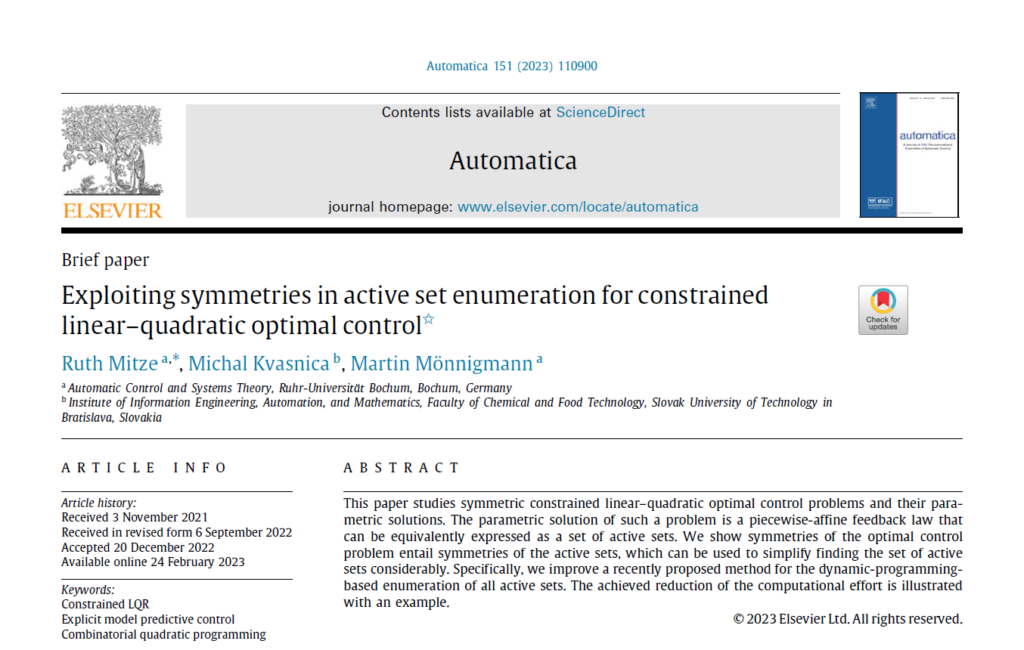
.
4. Heat exchanger control using model predictive control with constraint removal
(https://zenodo.org/records/7767419)
This paper, published in Applied Thermal Energy (Elsevier), focuses on enhancing the control of heat exchangers through Model Predictive Control (MPC) with constraint removal for improved computational efficiency. Heat exchangers are known for their high energy demands, making them challenging for advanced controller design. The complexity of real-time MPC implementation hinges on the number of inequality constraints in the optimization problem. Thus, to reduce computational effort, inactive constraints are removed. The paper proposes a modified constraint removal approach to ensure robustness suitable for practical applications, such as laboratory-scaled heat exchangers. The control performance is evaluated from an industrial standpoint, considering computational time and energy consumption, with the control approach implemented on a 32-bit microcontroller.
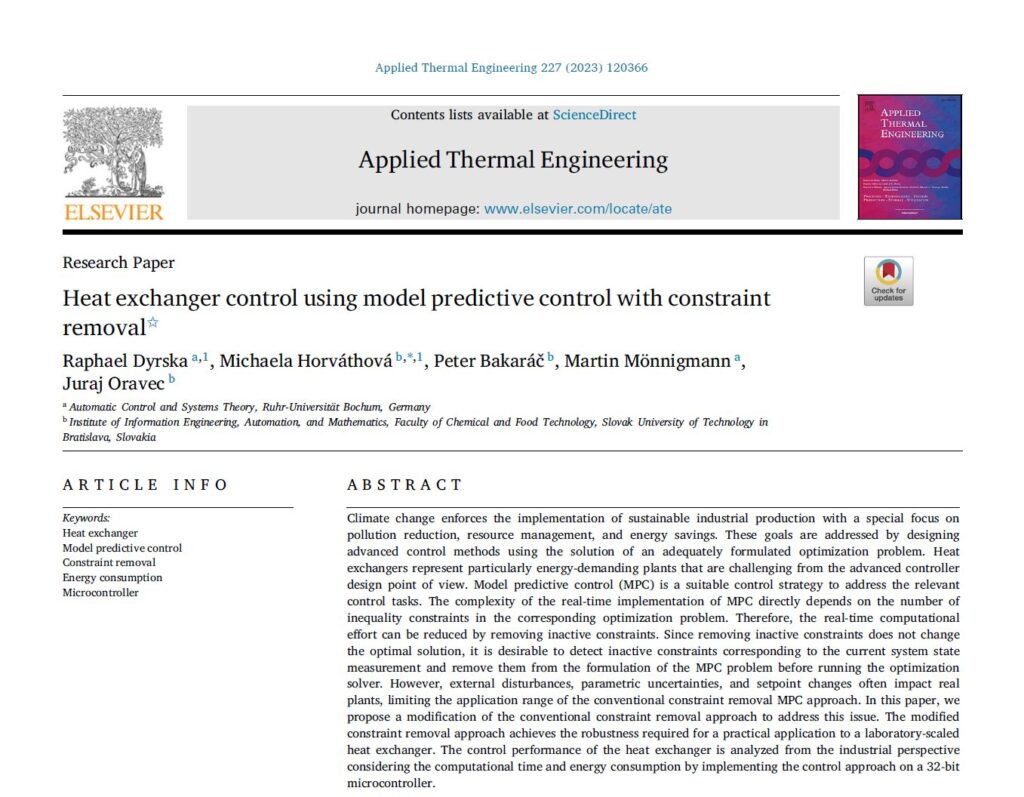
.
5. Data-driven models for a salt production process towards an Industry 4.0 evolution
(https://zenodo.org/records/10045329)
Presented at the IFAC World Congress 2023, this study focuses on developing a predictive model for the production process of brine salt at an industrial site in Italy. Utilizing standard statistical techniques for dimensionality reduction, the methodology involves acquiring one year of production data directly from the plant control system. A Python code is developed to analyze each plant, process raw data, and regress models using principal component regression (PCR) and partial least squares (PLS). While the results demonstrate reliable prediction for the evaporative plant, refinement is needed for the depuration model to enhance its performance.
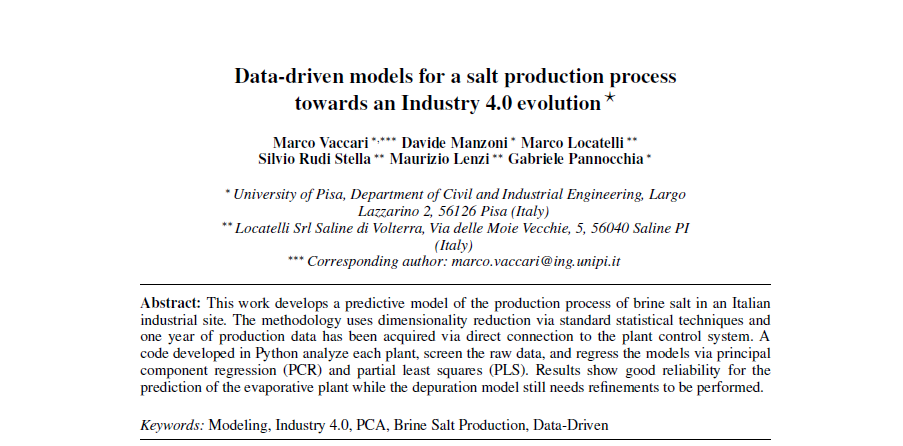
.
6. Easy-to-use MPC tool for controlling chemical processes in a rigorous simulation environment
(https://zenodo.org/records/8093913)
Presented at the IFAC World Congress 2023, this paper explores the use of rigorous process simulation to maximize profit, highlighting the superiority of detailed thermodynamic models over linearized ones. Customized Model Predictive Controllers (MPC) are introduced as effective tools for enhancing process performance compared to traditional control architectures. The paper presents an accessible MPC algorithm developed in Python, integrated with the UniSim Design® simulator. Communication via UniSim Design® spreadsheets enables Python to manipulate variables during simulation. Two test cases—a simple two-phase separator and a complex debutanizer column—illustrate the methodology’s effectiveness. System identification is employed to create linear models for the controllers, and various MPC designs are analyzed, producing satisfactory outcomes.
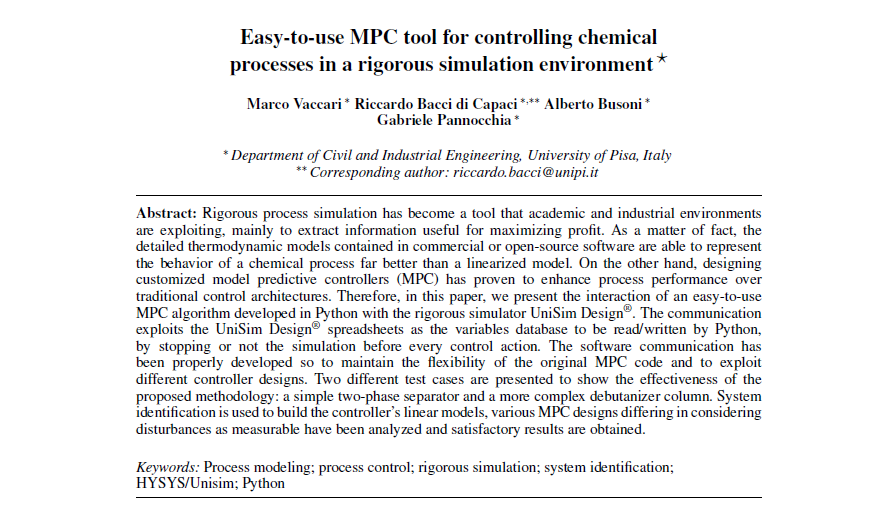
.
This project has received funding from the European Union’s Horizon under grant no. 101079342 (Fostering Opportunities Towards Slovak Excellence in Advanced Control for Smart Industries).
0 Comments