On December 8, we organized a scientific seminar on “On Symmetry in the Sequential-dependent Information Fusion in Neural Networks“. The scientific seminar was led by Mikel Ferrero Jaurrieta, a PhD researcher at Universidad Pública de Navarra, Spain. The scientific seminar was organized in the framework of the FrontSeat project as part of the seminar series on “Research Seminar on Smart Cybernetics”.
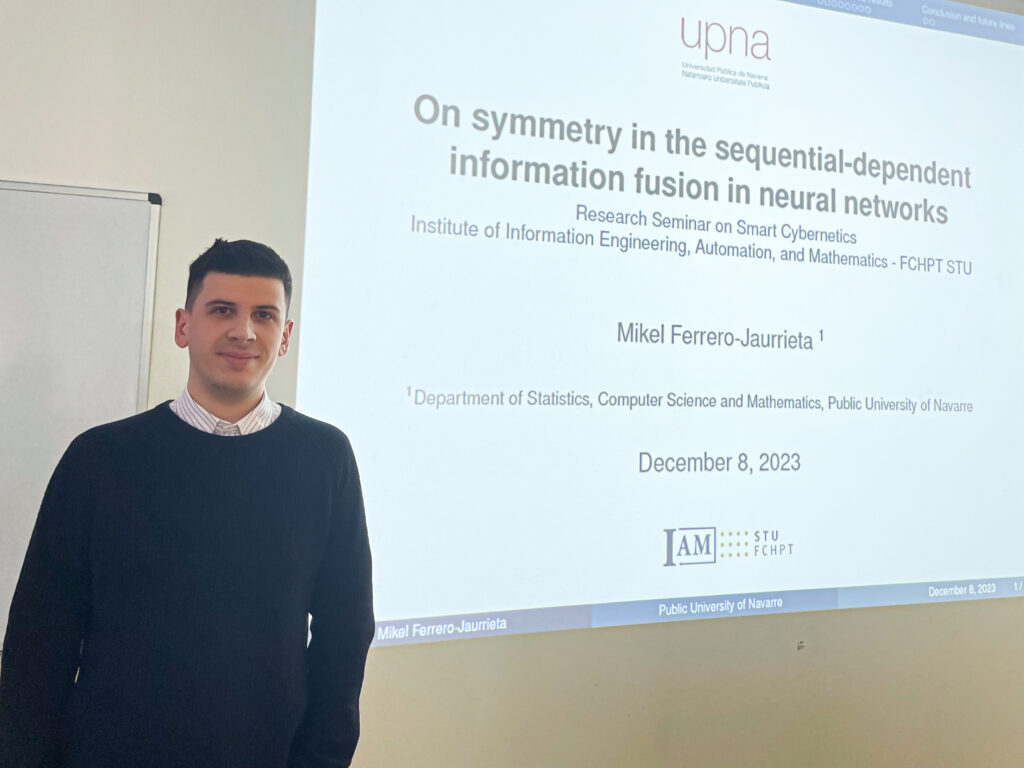
Abstract:
Data fusion in artificial intelligence systems is not a trivial issue. In the specific area of deep learning models, examples include pooling layers in Convolutional Neural Networks (where local features are fused to obtain a global feature), gates in Recurrent Neural Networks (where features that control the information flow of a network are fused) or attention mechanisms. However, the functions commonly used in the literature are symmetric when fusing features with sequential or temporal dependency, such as process mining, time series, text-based data, etc. The property of symmetry for a fusion function implies that the same result is obtained for different orderings of the same data collection. The order in which the information is merged is relevant when working with sequential information, where the data has time dependency. In this work, we discuss the necessity or not of the symmetry property as well as the comparison of results in some real cases with sequential information fusion in neural networks.
This project has received funding from the European Union’s Horizon under grant no. 101079342 (Fostering Opportunities Towards Slovak Excellence in Advanced Control for Smart Industries).
0 Comments