On May 27, we organized a scientific lecture on “From Data to Alarms: Data-driven Anomaly Detection Techniques in Industrial Settings”. The seminar was led by Rastislav Fáber, a PhD student at Slovak University of Technology in Bratislava. The talk concluded his visit to the Control Group at the University of Pisa.
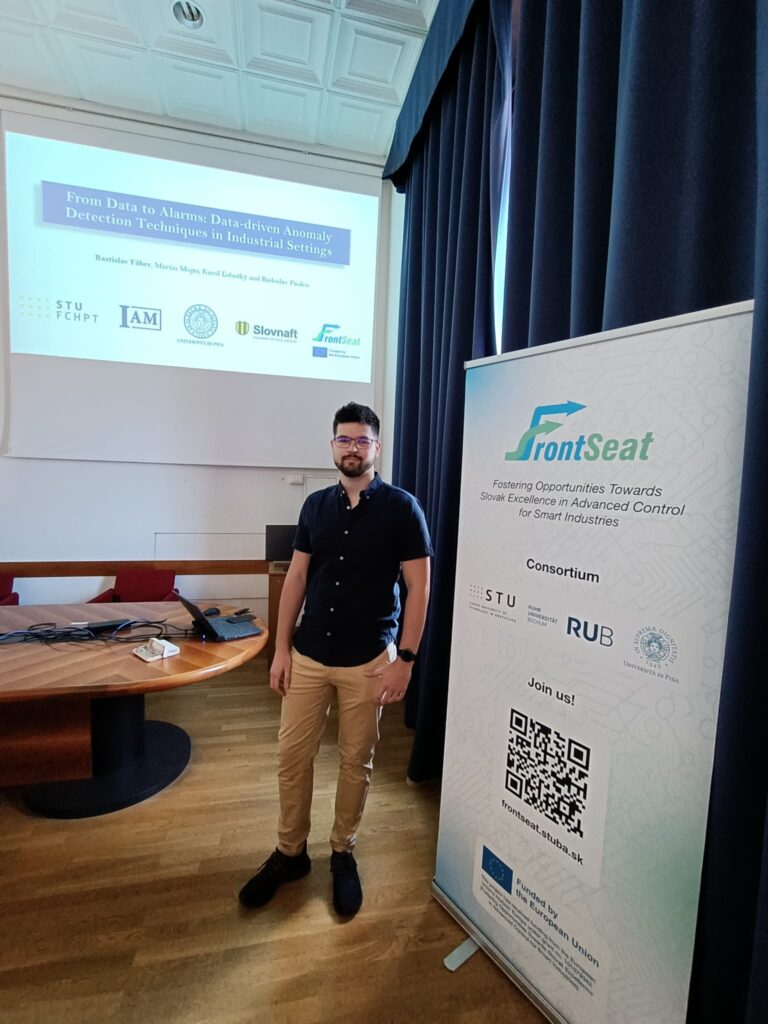
Abstract:
This presentation introduces a data-driven approach to anomaly detection in industrial processes, emphasizing the reduction of misclassifications during normal operations and improving the identification of anomalies and outliers. The optimization is guided by presumed ground truth (GT) labels linked to a dependent variable, specifically isobutane concentration. By employing a moving-horizon strategy on a large industrial dataset, we thoroughly evaluate various filtering algorithms and present a representative method for outlier classification. Additionally, we differentiate effective anomaly detection from outlier detection by incorporating a regression model. This model is trained using measurements from independent process variables to predict the dependent variable. Our results show that the trained regression models consistently provide accurate predictions, maintaining values within an acceptable process tolerance.
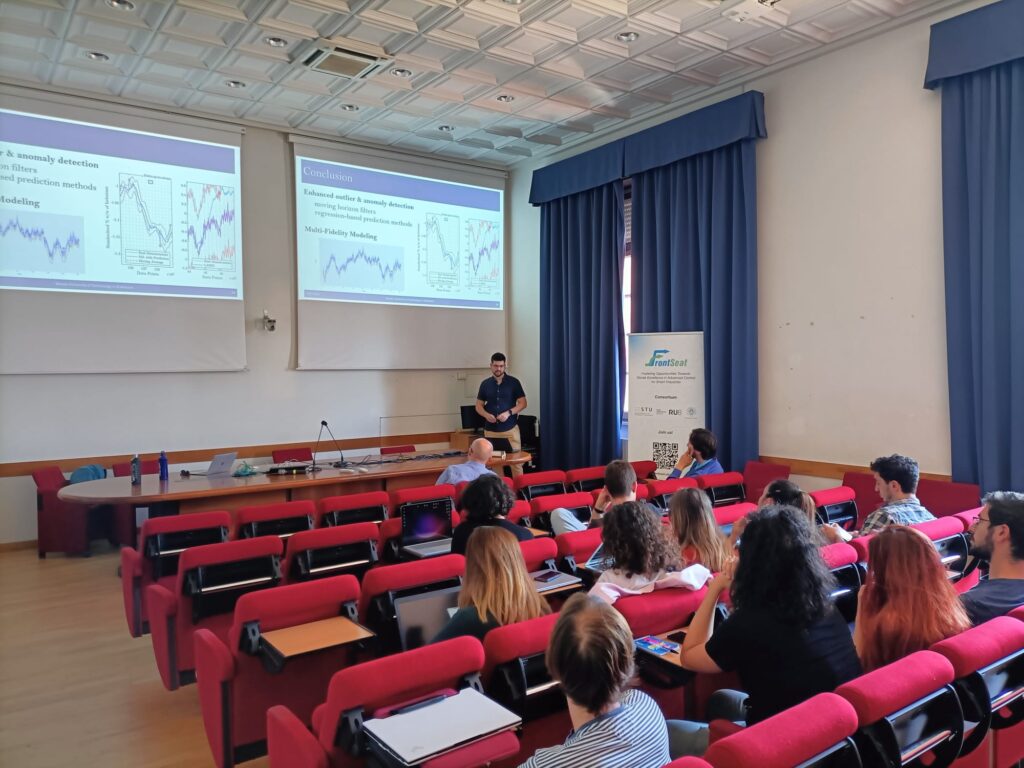
.
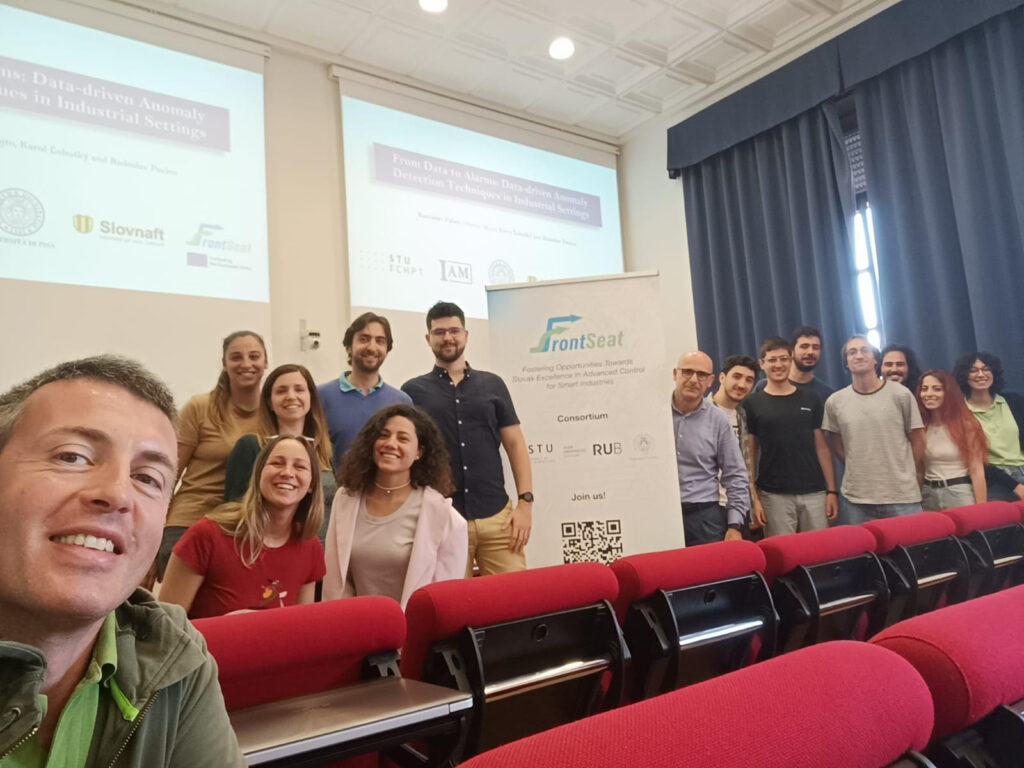
This project has received funding from the European Union’s Horizon under grant no. 101079342 (Fostering Opportunities Towards Slovak Excellence in Advanced Control for Smart Industries).
1 Comment
Research Exchange from STUBA to UNIPI - FrontSeat · June 20, 2024 at 1:14 pm
[…] my time at UNIPI, I presented my research on data-driven anomaly detectiontechniques in industrial settings, which was well-received by both faculty and students. Thispresentation was a significant […]