On May 12, we organized a scientific seminar on “Deep-Learning-Driven Tunable MPC with Stability Guarantees“. The scientific seminar was led by Michaela Horváthová, a PhD student at the Slovak University of Technology in Bratislava. The scientific seminar was organized in the framework of the FrontSeat project as part of the seminar series on “Research Seminar on Smart Cybernetics”.
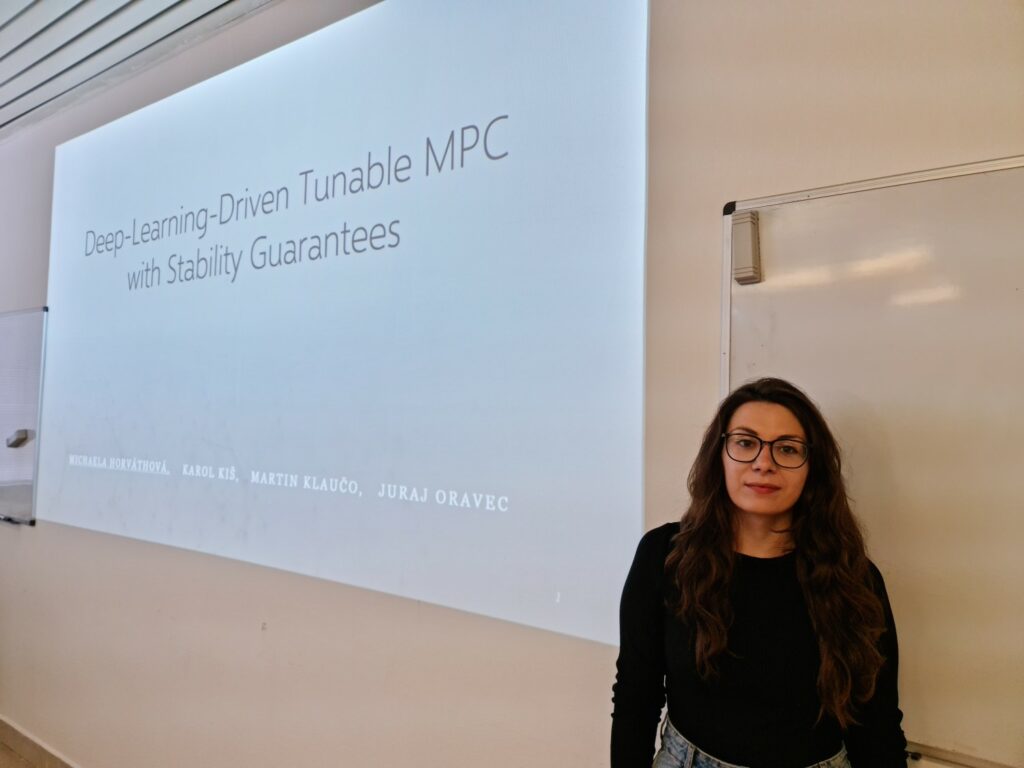
Abstract:
Safe real-time tunable approximation of the optimal controller enables its integration into industrial applications. Technically, we introduce a Neural-Network-based approximation of the optimal control law generated by a robust Model Predictive Control (MPC), preserving all the necessary properties of the MPC— the satisfaction of input and state physical constraints, recursive feasibility, and robust exponential stability. Moreover, we introduce the ability to tune this approximation in real-time while ensuring the satisfaction of input and state physical constraints, and the recursive feasibility of the real-time tuned controller. The real-time tunability could significantly improve the control performance of the devices with restricted computational and memory capacity. Compared to implicit MPC, the proposed method does not require any real-time optimization, and the approximated control law is suitable for verification/certification. Moreover, the implemented code is independent of external libraries. Compared to explicit MPC, the proposed method eliminates the necessity to solve (potentially time-consuming) evaluation of explicit solution map/piecewise affine function, i.e., removes the real-time point location problem, and, simultaneously, significantly reduces the memory footprint. The advantages of the proposed method are demonstrated by a double integrator benchmark and a quadrotor position control.
This project has received funding from the European Union’s Horizon under grant no. 101079342 (Fostering Opportunities Towards Slovak Excellence in Advanced Control for Smart Industries).
0 Comments