On January 16, we organized a scientific seminar on “Data-driven Control and Optimization with Kernel-based Learning“. Yuning Jiang, a postdoctoral researcher at École Polytechnique Fédérale de Lausanne, Switzerland, led the seminar, which was organized in the framework of the FrontSeat project as part of the seminar series on “Research Seminar on Smart Cybernetics.”
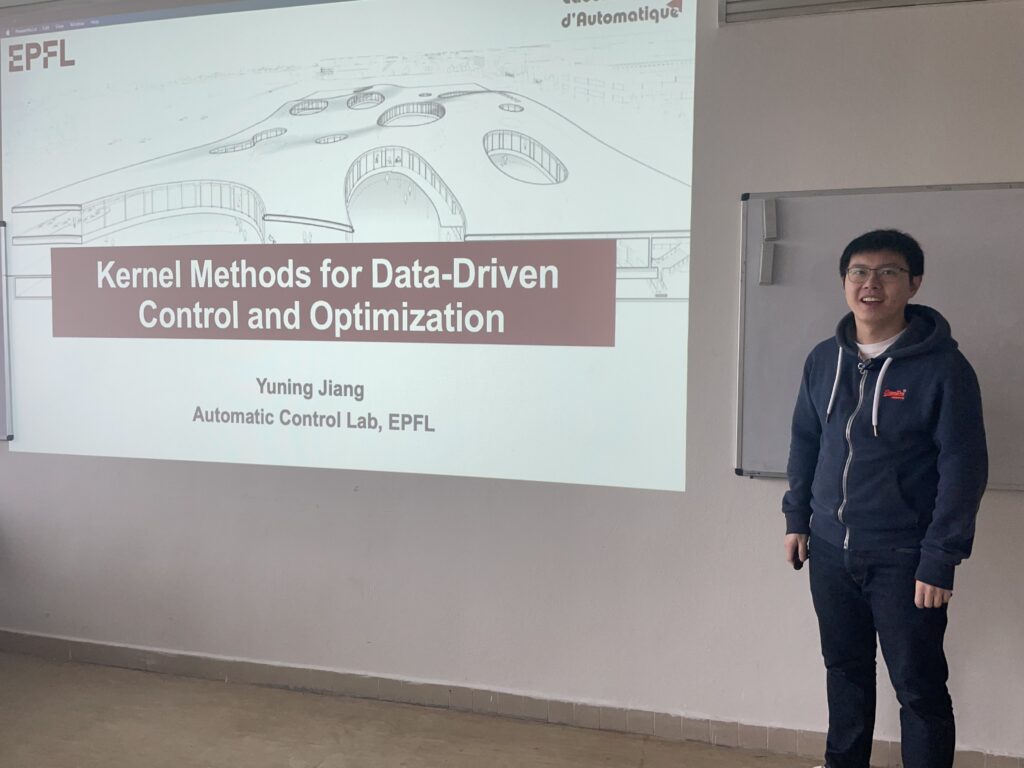
Abstract:
Kernel-based learning is a nonparametric learning technique widely used to interpolate an unknown nonlinear function based on a finite number of samples. This talk will first introduce the deterministic error bounds of the kernel models. These bounds could provide quantification of the state-dependently uncertainty for an unknown nonlinear dynamic system. Based on this advantage, the second part of this talk will present a data-driven predictive controller using Kernel-based system identification that enjoys deterministic guarantees of safety. Based on these bounds, the last part of this talk will introduce a black-box global optimization approach in RKHS with an explicit sublinear bound of the cumulative regret.
This project has received funding from the European Union’s Horizon under grant no. 101079342 (Fostering Opportunities Towards Slovak Excellence in Advanced Control for Smart Industries).
0 Comments