On April 10, 2025, we had the pleasure of organizing a scientific seminar as part of the Research Seminar on Smart Cybernetics series. Hosted at the University of Pisa and streamed online, the event provided a platform for knowledge exchange in the field of cybernetics and control systems.
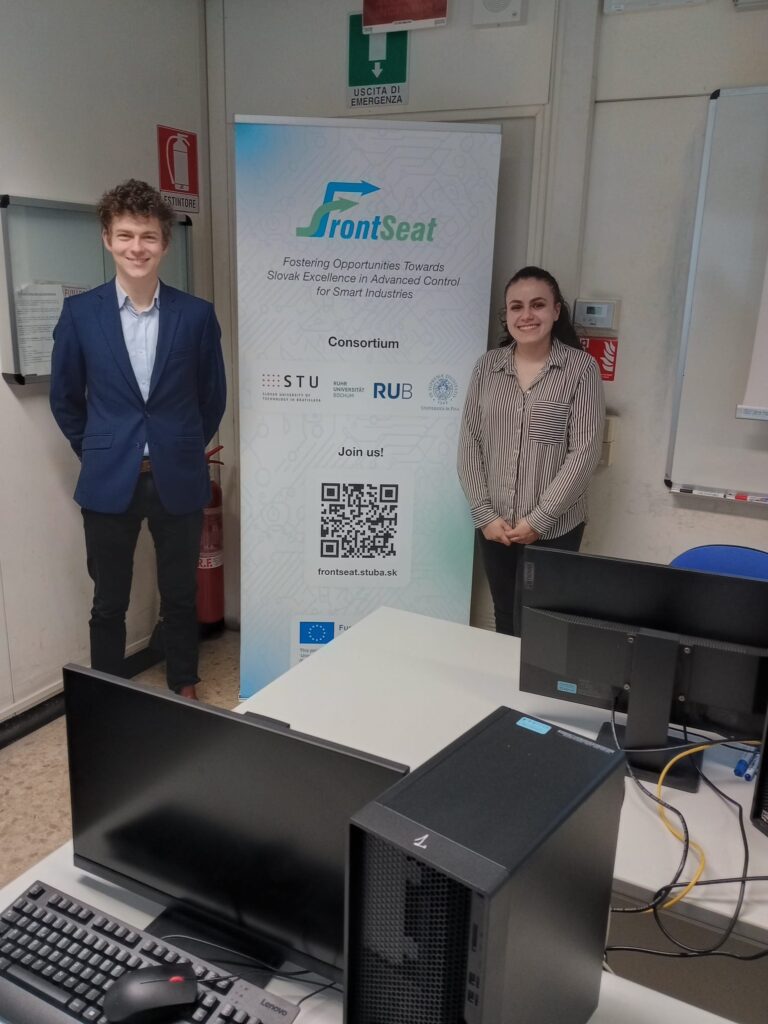
As part of the seminar, two PhD students from the Slovak University of Technology in Bratislava presented their latest research:
Koopman Model Predictive Control
by Patrik Valábek (Department of Information Engineering and Process Control, Faculty of Chemical and Food Technology, STUBA)
Abstract:
In recent years, the Koopman operator theory has emerged as a powerful framework for lifting nonlinear dynamical systems into a linear representation in a higher-dimensional space, enabling the application of linear control techniques such as Model Predictive Control (MPC). This seminar explores the application of Koopman-based MPC (K-MPC) to a pasteurization unit, showcasing how the Koopman operator can be used to derive a linear predictive control strategy for a nonlinear thermal process.
The first half of the talk will focus on implementing K-MPC on the pasteurization unit, covering the approximation of the Koopman operator, the construction of a linear predictor, and the formulation of the MPC problem. Special attention will be given to the specific transformations, design choices, and practical considerations necessary for successfully deploying a Koopman-based control on a physical plant.
The second half will introduce my ongoing research on offset-free Koopman MPC, which aims to extend the standard K-MPC formulation to handle steady-state tracking errors caused by model mismatch and disturbances. I will introduce the necessary transformations and modifications required to embed integral action into the lifted linear Koopman system, enabling offset-free tracking performance.
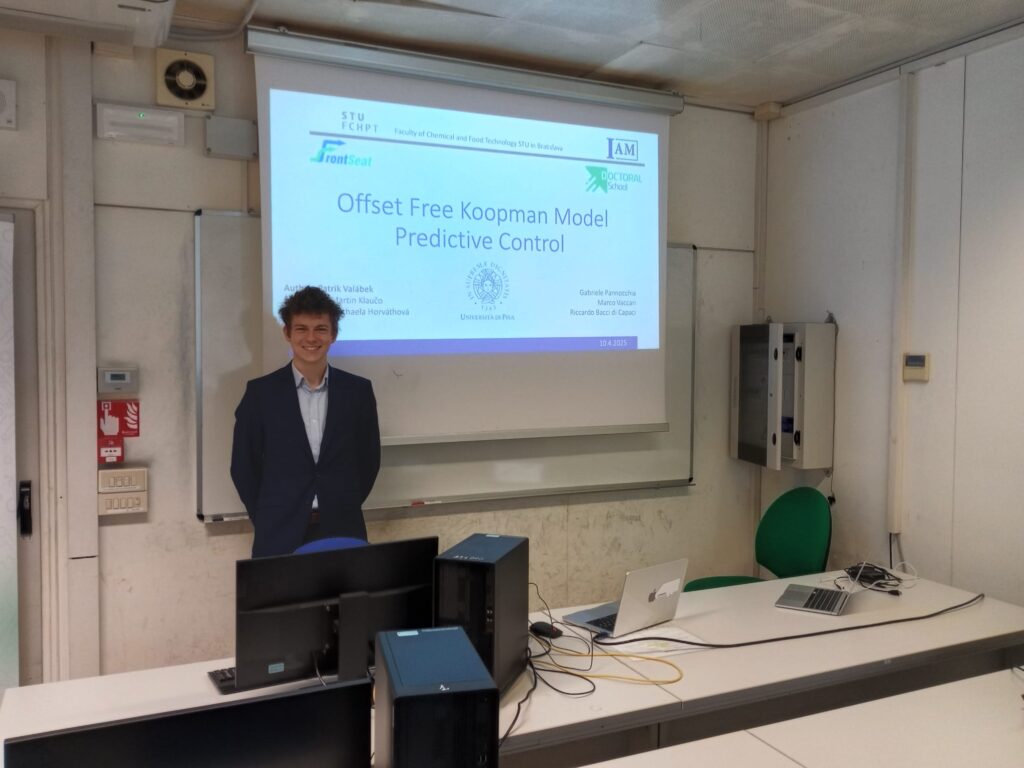
Towards Tunable Offset-Free Approximated Explicit MPC
by Erika Pavlovičová (Department of Information Engineering and Process Control, Faculty of Chemical and Food Technology, STUBA)
Abstract:
Real-time tunability is a known limitation in the application of explicit Model Predictive Control (MPC), as changes in weighting factors typically require a complete reconstruction of the parametric solution. This research project directly builds on the approach presented by Oravec and Klaučo (2022), where an asymptotically stable and recursively feasible real-time tunable approximated explicit MPC policy was proposed. In their method, nearly optimal control actions are generated through a convex combination of precomputed boundary explicit MPC controllers, enabling adaptation to changing performance priorities
without regenerating the entire explicit solution.
Building on this idea, the current research explores the extension of such a framework toward the offset-free control design problem. The methodology integrates multiparametric programming with a disturbance observer to ensure offset-free performance under changing reference values. A key challenge lies in adjusting the terminal set in response to reference variations while preserving theoretical guarantees such as stability and recursive feasibility. Preliminary strategies for terminal set tuning are presented alongside early simulation results for the offset-free explicit MPC formulation. The presentation outlines the current framework, discusses encountered challenges, and highlights perspective directions for ongoing research.
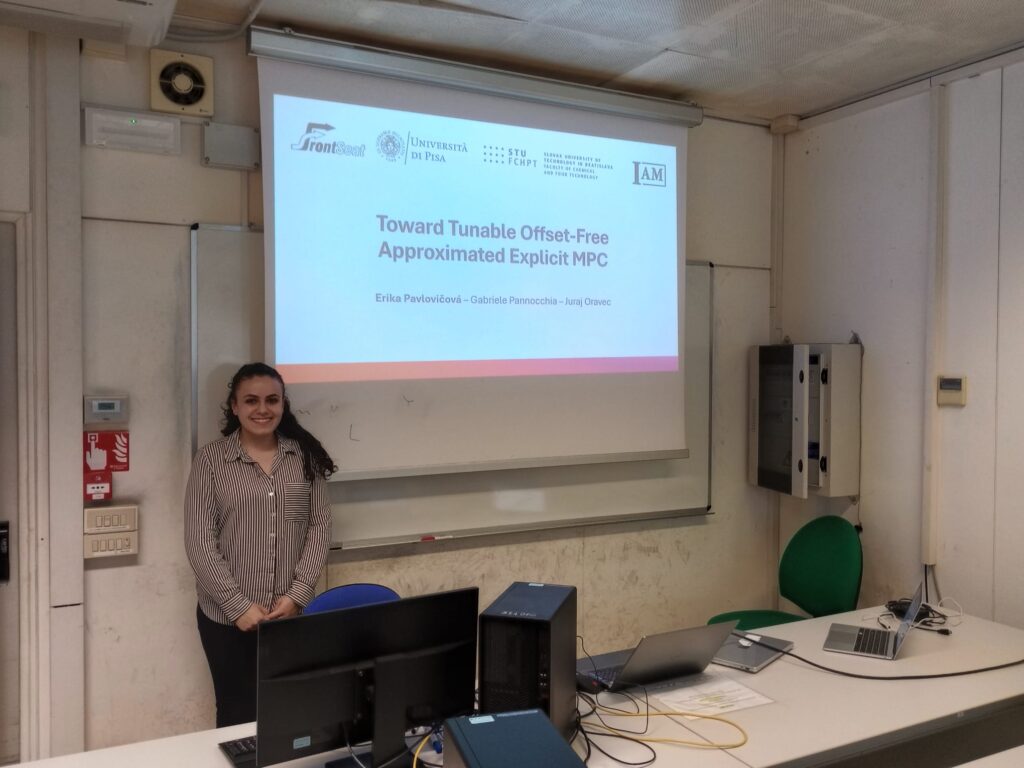
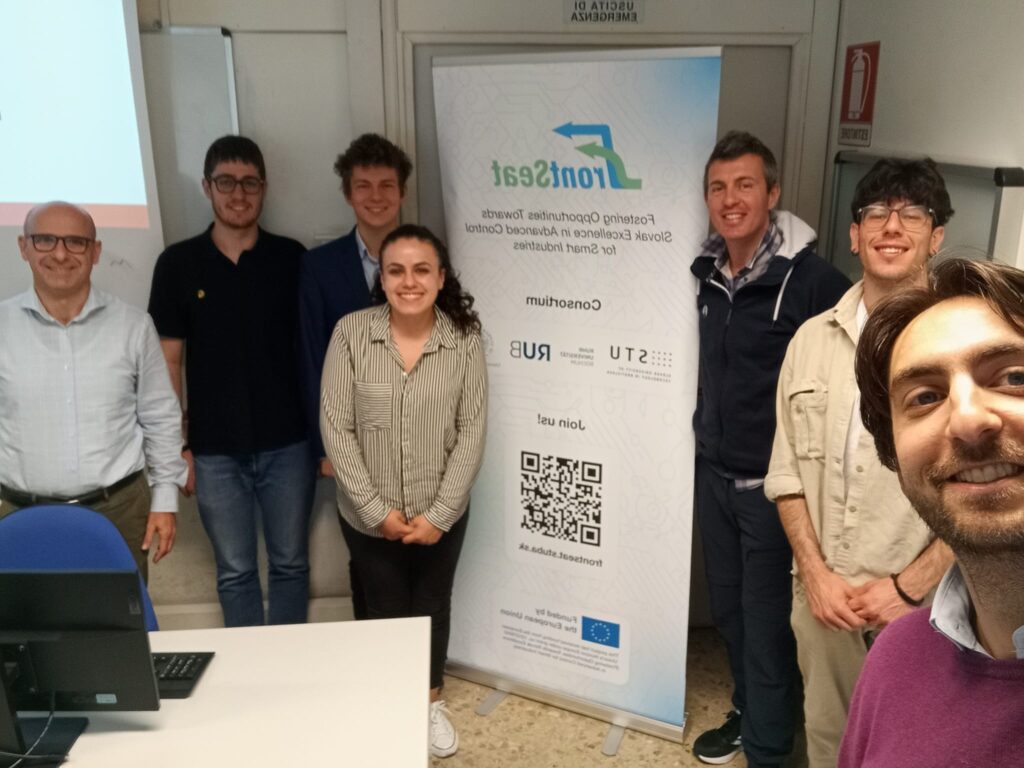
This project has received funding from the European Union’s Horizon under grant no. 101079342 (Fostering Opportunities Towards Slovak Excellence in Advanced Control for Smart Industries).
1 Comment
A Month in Pisa: Advancing Offset-Free Explicit MPC under the Tuscan Sun - FrontSeat · May 13, 2025 at 11:15 am
[…] and computational effort. I also had the chance to present my preliminary findings at a seminar, which opened valuable discussions and shaped the next phase of my […]